How digital public goods can help unlock the public interest potential of AI
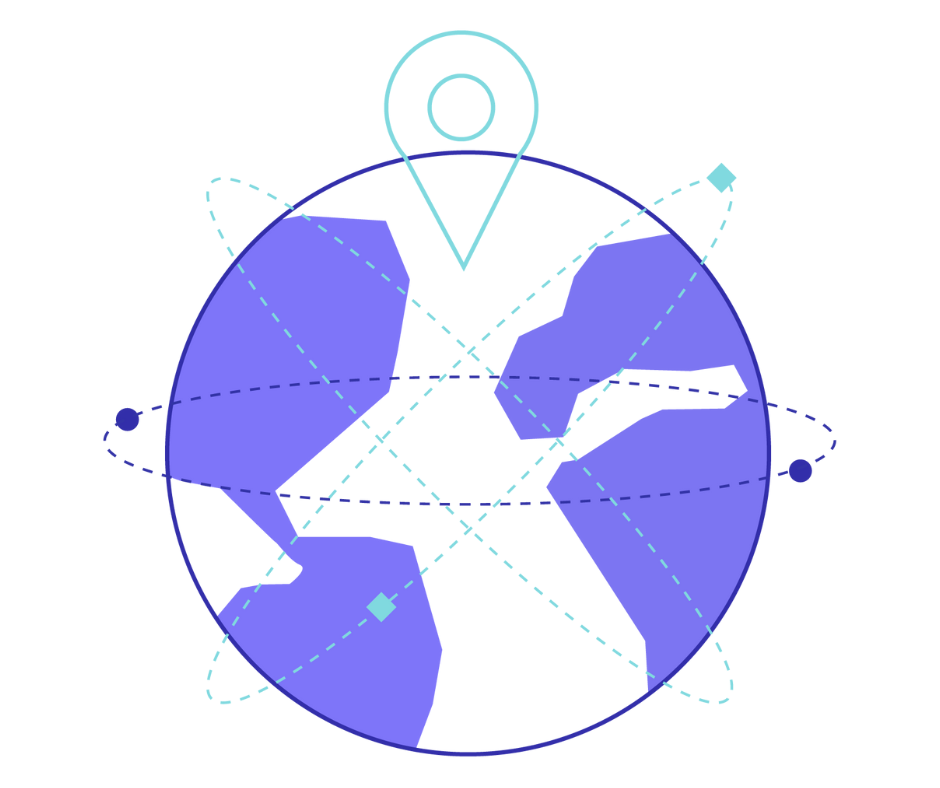
Author: Liv Marte Nordhaug, Secretariat CEO, Digital Public Goods Alliance
Over the course of the last few months, culminating with in-depth workshops at the recent 2024 Digital Public Goods Alliance Annual Members Meeting in Singapore, the DPGA Secretariat has spent a lot of time convening discussions on artificial intelligence (AI), in particular related to how digital public goods can advance public interest AI. Throughout these discussions, participants have highlighted multiple challenges that are preventing the advancement of public interest AI at scale. This is why in 2025 we want to help source open-source tools that can reduce these hurdles and be part of the solutions needed.
While there is no precise agreement on the definition of public interest AI, the generally accepted understanding, as mentioned in a June DPGA Secretariat blog, includes the following: “desired objectives such as better enabling the use of AI to tackle urgent social and environmental challenges, improving access to AI development capacities to spur innovation and foster the creation of localised solutions for context-specific challenges, supporting basic AI research and research in other fields such as drug development, and shaping market structures to address market imbalances”.
With this in mind, it feels natural that digital public goods (DPGs) should play a strong role in the pursuit of public interest AI, but that’s not to say it will be straightforward. As I previously highlighted in another DPGA blog, “maintaining a high bar on training data could potentially result in fewer AI systems meeting the DPG Standard criteria. However, SDG relevance, platform independence, and do-no-harm by design are features that set DPGs apart from other open source solutions—and for those reasons, the inclusion of training data is needed”.
In the same blog I also wrote that “with DPGs, we want to help evolve the public interest AI landscape as the ecosystem gains a better understanding of how to address complexities regarding open data and data sharing”.
At the DPGA Secretariat we have continued to ask ourselves and relevant experts how we can help move the needle on some of these complexities. Here, I highlight where our thinking currently stands:
DPGs as tools for public interest AI
We would like to fast-track the use of DPGs that can serve as tools for addressing the barriers to advancing public interest AI. This could for instance include solutions for improving data governance, transparency and accountability; consent and licensing for training; and regulatory compliance and policy priorities. We will work to surface such open source tools while remaining fully committed to advancing AI systems as DPGs, where each relevant component of a given AI system (including the training data) is made openly available and other DPG-relevant criteria are met.
Some barriers are purely technological, whereas others relate to established processes and norms, including a need to build awareness, knowledge and trust. In some cases, legislative changes or other forms of legal procedures are needed before meaningful action can be taken, whereas other challenges could be addressed right away – with the right tools. Here are some examples of technical challenges or topics we have heard mentioned so far, where DPGs could potentially be of use:
- Extracting data from non-machine-readable formats (such as PDF).
- Identifying licensing information, public domain status or consent signals of content/data.
- Data provenance tracking.
- Testing and validation datasets.
- Collection and labelling of data (such as multilingual data).
- Synthetic data generation, anonymization and masking.
DPGs, as open, adaptable digital solutions, with documentation that can help facilitate reuse, can play an important role as tools for addressing common challenges to scaling public interest AI – both in the near future and longer term. In particular DPGs can help unlock more and higher-quality open training data and data sharing. They can also address other public interest AI challenges such as the testing and validation of AI systems, and potentially provide tools, resources, or serve as examples for how to lower the computing power requirements for AI development and deployment, making AI more accessible in resource-constrained environments and reducing the energy footprint.
Ideally, we would like to see the development of a co-evolved toolkit of complementary open source tools that many stakeholders can use and adapt as needed to address their specific or unique challenges. Success in identifying and/or building the most high-impact DPGs as part of the toolkit will depend on mobilizing diverse groups of experts and stakeholders committed to advancing public interest AI to collaborate. We believe that focusing on use cases will be important for these efforts.
High-impact use cases
The DPGA Secretariat received helpful recommendations and insights on where the greatest opportunities for alignment are during the three different public interest AI-sessions at the DPGA’s Annual Members Meeting in Singapore.
One point of consensus was that while there are highly complex challenges pertaining to open data and data sharing, particularly when significant privacy considerations and sensitive personal data is involved, there are other sectors that can be addressed in a more straightforward manner. For example, areas that largely do not involve personally identifiable data, like satellite imagery, open climate and nature science, and supply chain information may be more straightforward to advance and valuable for public interest AI and the sustainable development goals.
Another reflection shared was that while many types of data collection could bring privacy risks, for instance voice data used for developing large language models, many privacy risks can be addressed if there are well designed privacy preserving collection and management processes in place.
Lastly, participants gave several examples of the need to build confidence among public sector officials in how AI can be used in a safe way to improve their public service offerings. Starting small by using existing open data from these institutions to train a small language model to address a specific public service need could be a way of helping address this concern and foster positive change and trust in AI.
Informed by these and other discussions we have arrived at the following reference use cases for where DPGs should be identified and/or built as tools for more high-impact public interest AI:
- Multilingual large language models (LLMs) covering underserved languages,
- Small language models (SLMs) that can address more specific needs, particularly in public service delivery,
- Research-based climate action (monitoring, mitigation, adaptation).
We believe these use cases are well aligned with the DPGA Vision of advancing the sustainable development goals and contributing to a more equitable world. Importantly, as we refine these topics further, we will ensure that our understanding of pressing needs and challenges in relation to each use case continues to be informed by stakeholders from low- and middle-income countries, including from among the DPGA membership.
We will launch a process for creating this toolkit in late February 2025, and hope you will join us in this journey!