Toward public interest AI: the role of AI DPGs and public resources for AI
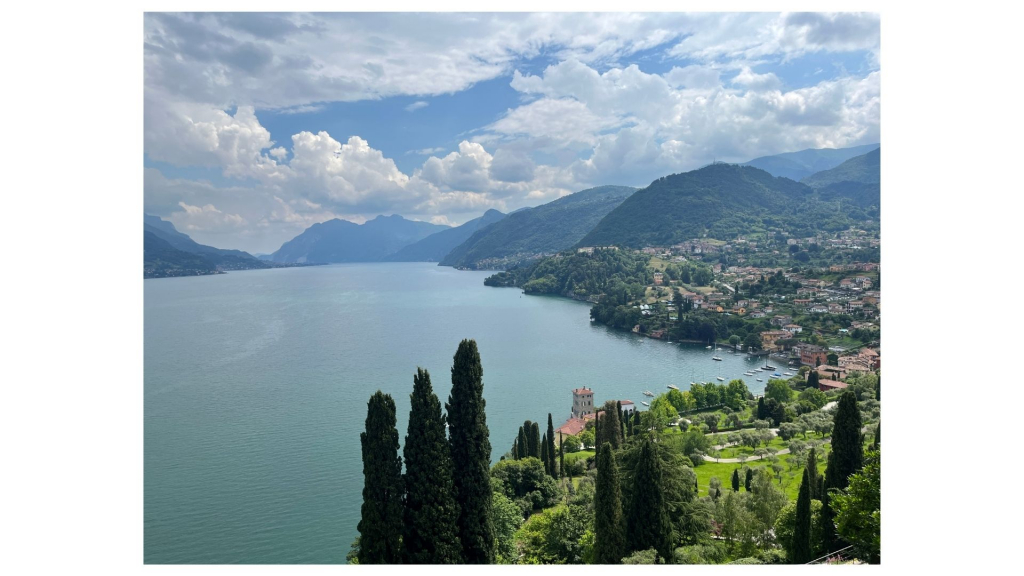
Author: Lea Gimpel, Director of AI and Country Engagement (Visiting Fellow)
The rise of public interest AI, which broadly refers to AI systems that serve the long-term survival and well-being of humankind, and public resources for AI, which includes infrastructure and funding to AI developers and researchers, can potentially boost the development of innovative AI solutions that tackle urgent global challenges ranging from climate change to healthcare. Although technically separate from one another, both public interest AI and public resources intersect as they can accelerate the development, deployment, and inclusive governance of AI DPGs (1) —AI systems that are open-source (2), SDG-relevant, and do no harm by design. The following post reflects on this intersection between public interest AI, AI DPGs, and public funding for AI infrastructure. This piece was informed by the Bellagio Convening on Public Resources for AI held by the Rockefeller Foundation between June 3 and 7, 2024.
Ever since OpenAI launched ChatGPT, discussions regarding AI regulations have intensified among policymakers, technologists, and academics. This has resulted in tangible outcomes such as the EU AI Act and the US Executive Order on Safe, Secure, and Trustworthy Development and Use of Artificial Intelligence. Alongside the strong interest in AI regulation, there has been a recent shift towards questions related to public interest AI to ensure advancements in AI technologies are human-centred and serve the public good. This is shifting the conversation to move beyond discussing how to mitigate risks, as reflected in the aforementioned regulations. Concurrently, the term “public AI” emerged, referring to AI as public infrastructure that is made accessible by the public sector to benefit all, is publicly accountable and reflects society’s values.
Although there is no consensus, several desired objectives tied to the public interest and public AI include:
- better enabling the use of AI to tackle urgent social and environmental challenges,
- improving access to AI development capacities to spur innovation and foster the creation of localised solutions for context-specific challenges,
- supporting basic AI research and research in other fields such as drug development,
- shaping market structures to address market imbalances
Currently, market dominance throughout the AI technology stack (i.e., hardware, compute, data sets, models, benchmarks, and other tools) is highly centralised and dominated by very few companies and regions. This limits AI’s potential public interest benefits and worsens structural inequalities within and between societies because most countries lack access to critical AI development resources. This is particularly concerning as many such countries are disproportionately facing market failures in delivering basic services. There is currently no market opportunity for big tech companies that would justify private investments in relevant local, domain-specific datasets, model training, and product development that could address development challenges. Therefore, local solutions are needed.
To shift the current problem, access to foundational AI infrastructure components needs to be increased. Several initiatives are underway, such as The National AI Research Resource in the US, and the European Union’s High-Performance Computing Joint Undertaking and their collaboration with African partners. However, access to compute is just one aspect and comes with additional questions regarding funding and building sovereign compute infrastructure. Other layers of the AI technology stack, such as data sets, models, and benchmarks, also must be addressed (3). Ideally, this is done in an open-source way to facilitate equitable access and representation. Without such interventions, most countries will continue to face enormous hurdles to develop high-impact public interest AI solutions that could address local development challenges. Thus, public-interest AI necessitates not only meaningful regulation to prevent harm but also the allocation of public resources across the AI technology stack to accelerate public benefits beyond private market interests. This also stands in the tradition of previous public investments in technology to benefit society, such as the invention of the internet and early missions to space.
Several organisations do fantastic work at the intersection of these debates, including, but not limited to the AI Now Institute, the Economic Security Project, Open Future, The Collective Intelligence Project and Coding Rights (4).
Yet, much of this requires nuanced discussions around means and ends, a deeper understanding of the AI technology stack and the generalizability of its components, but also normative principles to guide any public investments in the AI technology stack for public benefit. From the perspective of the Digital Public Goods Alliance Secretariat and our work on supporting the development, deployment and discoverability of AI DPGs, the following points highlight key considerations:
- Understanding the intersection of public interest AI and digital public goods: AI DPGs can play an essential role in ensuring that SDG-relevant open-source AI models or systems are available globally. They give the public and private sector the tools to advance public interest by using vetted open-source systems in the form of recognized AI DPGs that are designed to address social and environmental challenges. In a nutshell, all AI DPGs can serve the public interest if applied and governed responsibly, but not all public interest AI technology will be a digital public good because of limitations in their openness (see next point).
- Public interest AI is not open-source per se: AI that serves the public good should ideally be open-source and adhere to the DPG Standard to ensure responsible development practices (5). However, there might be several reasons why full openness is not desirable. These include, but are not limited to, the data subject’s rights to privacy, and trade-offs between public benefit and commercial interests.
- Public interest AI should favour small, task-specific models: To serve local communities by addressing specific use cases and enabling appropriate representation, eliminate bias stemming from large, unstructured datasets and mitigate environmental challenges stemming from the training of ever-larger models underpinning generative AI, focus should be laid on small, task-specific models. Given the lack of openness in generative AI models, which currently serve as a basis for many applications, such an approach would also support an increase in AI DPGs, given that smaller, responsibly developed models can comply more easily with the open-source definition and the DPG Standard.
- Investments along the AI technology stack to advance public interest AI should be in support of open-source AI: Public investments should enable open access to AI development resources without or with very limited gatekeeping, targeting and benefiting specifically underserved communities, researchers and SMEs. As the saying goes for software: “public money, public code”. The same should be true for any publicly funded development of AI systems to the biggest extent possible. If companies are built based on public funding, the public should hold controlling shares.
- Public investments along the AI technology stack should take a DPI approach: They need to be strategic and maximise outcomes by learning from implementations of digital public infrastructure. This means supporting platforms and building blocks rather than individual solutions, use cases, or organisations. Public investments in AI infrastructure should consider DPI architecture principles such as interoperability, multi-modal access, decentralised/federated infrastructure, and safety and security by design.
- Principles and conditions for investing public resources in AI are needed: These guidelines direct the supply of and access to public resources across the AI technology stack and should be developed in a collaborative, multi-stakeholder process. This includes ensuring that resources are accessible and empower disadvantaged groups, that outcomes align with sustainability considerations, focus on transformational impact, and more.
- Public investments in AI infrastructure can become a vehicle for democratic AI: Access to AI development resources by favouring an open-source approach is just one dimension of democratising and gearing AI’s development towards the public interest. By linking open-source communities with democracy activists, public AI infrastructure funding can become a force for collective development beyond open-source communities and inclusive governance.
Footnotes
1. The DPGA has been co-hosting a community of practice with UNICEF to define AI DPGs and develop recommendations for updating the DPG Standard. The recommendations will be made public in fall 2024, when the Open Source Initiative released its open-source AI definition. The interim report can be found here and a blog post outlining the progress made here.
2. Understanding that there is no agreed-upon definition for open-source AI systems, the Open Source Initiative (OSI) is currently facilitating an open, collaborative process to define it. The latest draft version can be found here.
3. Examples include the crowd-sourcing project for voice data “Mozilla Common Voice”, the indigenous data storage infrastructure built by Iwi Māori, for Iwi Māori and the SEA-LION family of LLMs developed by the Singaporean government.
4. Coding Right’s forthcoming report “AI Commons: nourishing alternatives to Big Tech monoculture” identified more than 200 organisations, many of which are based in global majority countries, that work towards a decentralised AI Commons that favours an alternative, equitable pathway to AI development. See also: https://codingrights.org/docs/Federated_AI_Commons_ecosystem_T20Policybriefing.pdf
5. The DPG Standard does not monitor downstream implementation of products recognized as digital public goods. Regardless, deployment, monitoring and governance of any AI DPG must follow ethical and responsible practices to serve the public interest.